Research topics
HAIF will establish a novel doctoral training scheme that connects University of Turku’s Faculties of Technology, Social Sciences, Law, Humanities and Medicine. By bringing together 15 University of Turku professors and 11 research groups and topics, HAIF creates a scientific community with unprecedented interdisciplinary knowledge and global partner networks.
HAIF doctoral researchers will perform novel research under scientifically distinguished and experienced supervisors. HAIF doctoral researchers get access to world-class research infrastructure such as high-performance computing offered by the Finnish CSC – IT Center for Science Ltd.
Below you can find a short description of each participating research group and the potential dissertation topics they offer.
Health Technology Research Group
AI Ethics and Issues of Responsibility
Materials Informatics Laboratory
Materials in Health Technology
Algorithmics and Computational Intelligence (ACI) Laboratory
Turku Natural Language Processing Group (TurkuNLP) – Computer Science
Turku Natural Language Processing Group (TurkuNLP) – Digital Linguistics
Health Technology Research Group
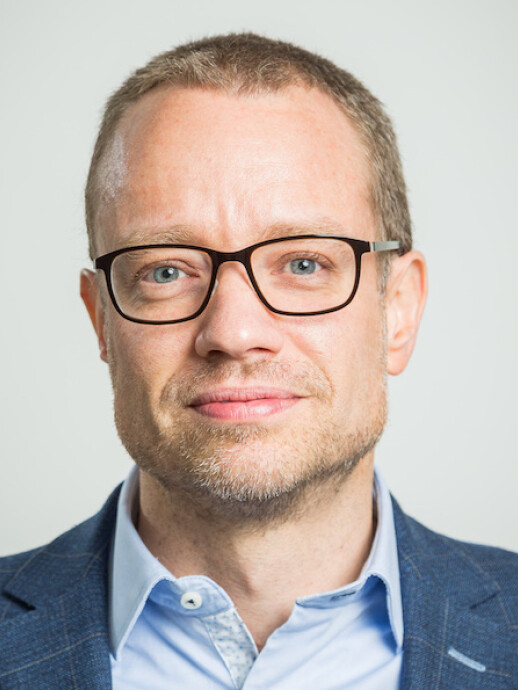
Pasi Liljeberg
Prof.
Group leader (PI) Prof. Pasi Liljeberg
Health technology research unit stands at the intersection of technology and medicine. We develop data-analytics based solutions to support health and well-being that can serve both medical professionals and the public. Our research focuses on human sensing solutions, wearable technologies, and machine learning based data analytics methods to help collect and utilise data in a user-friendly, safe, and cost-effective manner. Like many other fields of science, healthcare sector is moving towards data as the foundation of its decision making. The group leverages its research for developing AI based applications utilising health data for preventive and personalised healthcare.
Potential dissertation topics:
LLM-powered Health monitoring:
This research focuses on advance research in LLM-powered health monitoring. This will be done via developing innovative methods and frameworks leveraging large language models (LLMs) to interact with wearable devices, health data resources, and analytical tools such as biosignal processing and machine learning models. The aim is to enable more interactive and effective health monitoring, with applications in areas such as mental and maternal health.
Trustworthy Non-contact Biosignal Monitoring for Improving Maternal Health:
The health of pregnant women and newborn babies can be monitored via biosignals. These signals serve as indicators of maternal well-being, fetal health, and potential complications, such as stress, hypoxia, and cardiovascular issues, which can adversely affect pregnancy outcomes. The research explore non-contact methods for the measurement of heart rate (HR), heart rate variation (HRV), and oxygen levels in the blood (SpO2). Remote Photoplethysmography (rPPG) is a non-contact method for monitoring physiological signals using video recording of the subject’s face by utilising machine learning methods. By analyzing the subtle colour changes in the skin due to blood flow, rPPG enables the estimation of HR, HRV, and SpO2 without the need for physical sensors. Despite its potential, this method faces many challenges in practical use. The goal of this proposal is to build a trustworthy method for monitoring the health of pregnant women and newborn babies using rPPG.
Human sensing via wearable technologies:
The research focuses on utilising wearable technologies for human sensing solutions. This includes biosignal analysis, data science, and machine learning for developing and applying advanced machine-learning techniques to analyze complex physiological signals such as photoplethysmography (PPG), electrocardiography (ECG), and accelerometer data. The focus will be on leveraging advanced techniques to extract meaningful patterns, improve signal quality, and provide actionable insights for health monitoring and clinical applications.
Measuring Haemoglobin without Needles: A non-contact method for Haemoglobin level estimation:
The accurate measurement of haemoglobin levels is critical for diagnosing anaemia, thalassemia and polycythemia. Traditional methods, while reliable, involve the invasive procedure of drawing blood from the subject, which can be inconvenient and painful. We aim to develop a non-contact method, point-of-care solution, for haemoglobin level estimation using remote photoplethysmography (rPPG). The rPPG is a non-contact method for monitoring physiological signals using a video recording of the subject’s face.
Keywords: Health technology, biosignal analysis, large language models, machine learning, maternal health
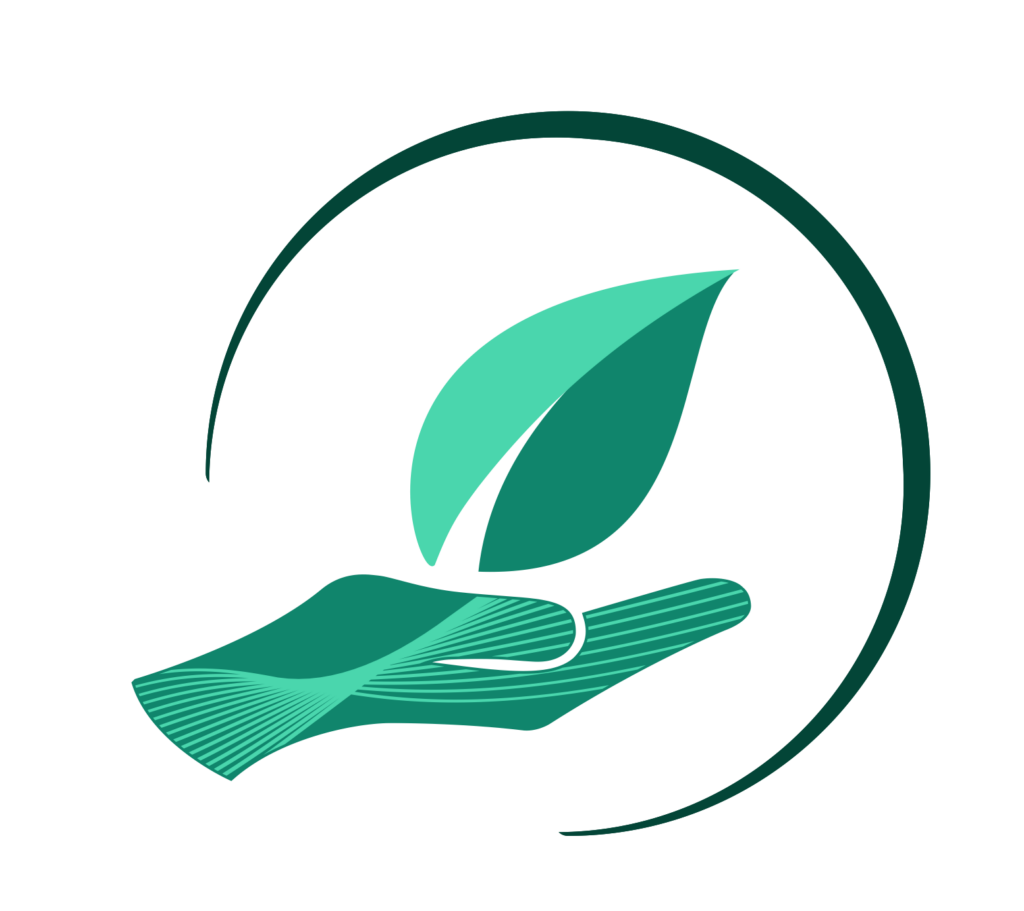
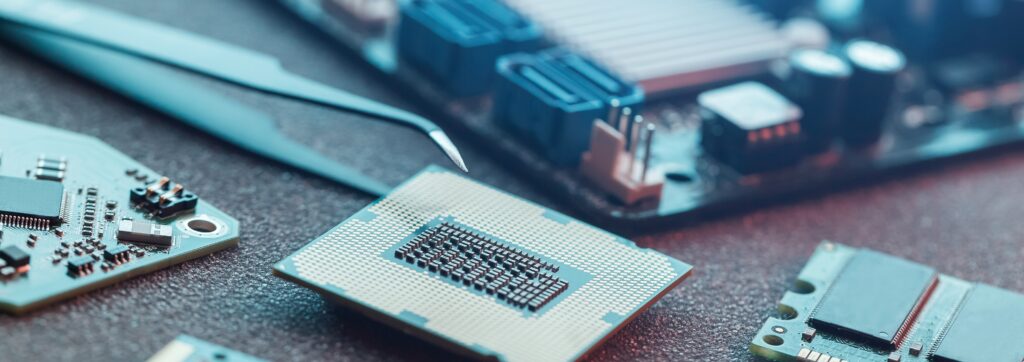
Autonomous Systems Laboratory (ASL)
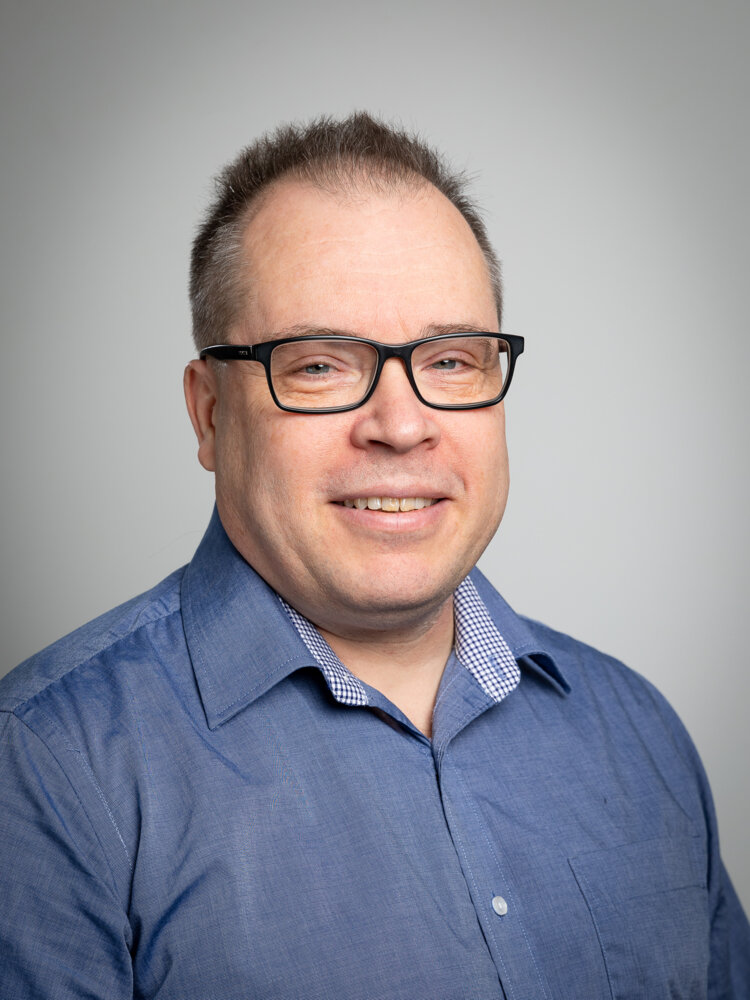
Juha Plosila
Prof.
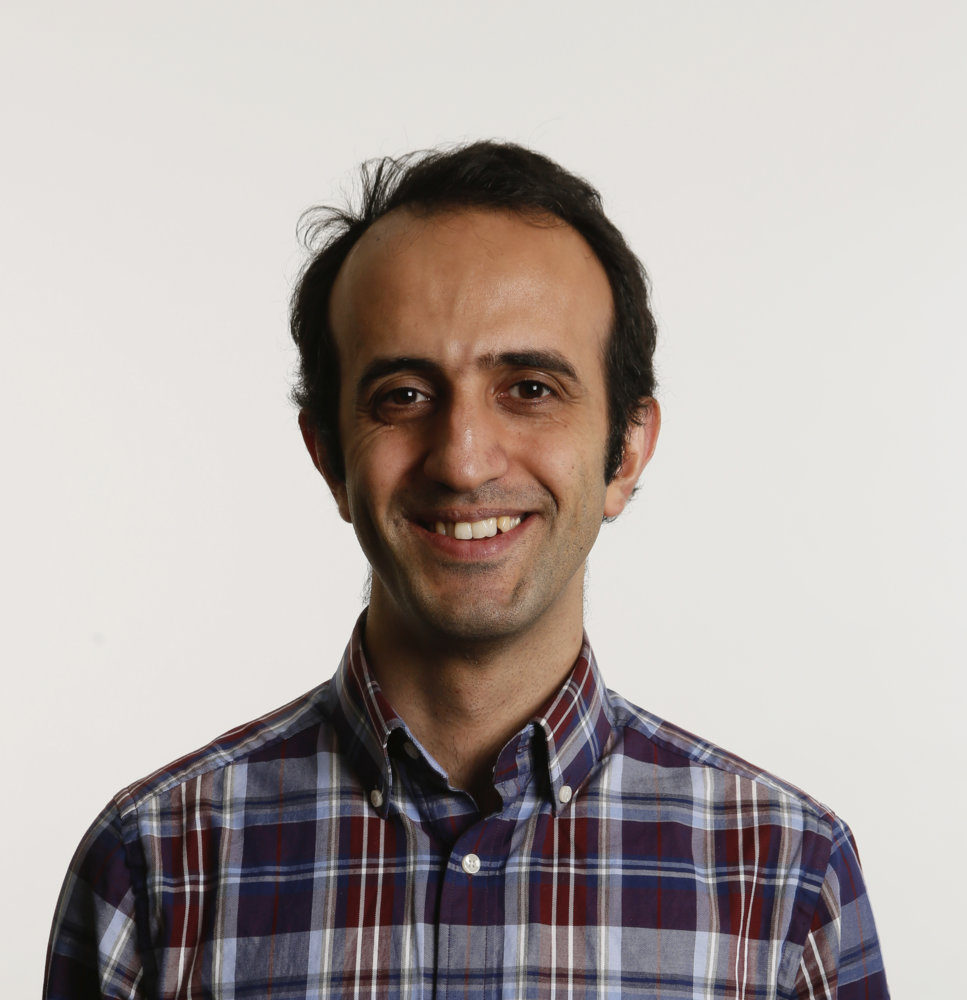
Hashem Haghbayan
Senior Research Fellow
Group leaders (PIs) Prof. Juha Plosila, Senior Research Fellow Hashem Haghbayan
Our research group focuses on the efficient co-adaptation of autonomous systems with humans, society, and the environment. This requires a mutual understanding of goals and the development of knowledge from both AI and human agents. Decisions made by AI agents must be transparent and comprehensible to humans, which can be achieved, for example, through methods of explainable AI and knowledge abstraction. Conversely, human information and objectives should be communicated seamlessly to AI agents through different channels, including natural language, patterns of human behaviour, and human-AI interactive signalling. The research group explores and develops various dynamic co-adaptation methods while also considering essential factors such as energy consumption, lifespan of autonomous systems, and the transparency and explainability of embodied intelligence in these systems.
Potential dissertation topics:
Active Learning of Human Behavior and Environmental Dynamics in Autonomous Systems
The aim of this project is to analyze, model, and predict the human environment, including human behavior, through active AI learning. The objective is to establish a knowledge structure for AI, such as a generative model, that can actively learn significant features of humans and their environment within a human-AI system, enabling predictions and modeling in both short term and long term. Key focus areas of the project comprise analyzing human body signals, modeling environmental dynamics, understanding AI-human reciprocal dynamics, and exploring active learning. The detailed research plan for a selected candidate will be developed based on these critical aspects of the project and the candidate’s own interests. Applicants should have a strong background in AI and statistical analysis, as well as proficiency in programming with Python and MATLAB. Experience with common AI frameworks, such as PyTorch, is essential. Knowledge of hardware for AI and robotics is a plus.
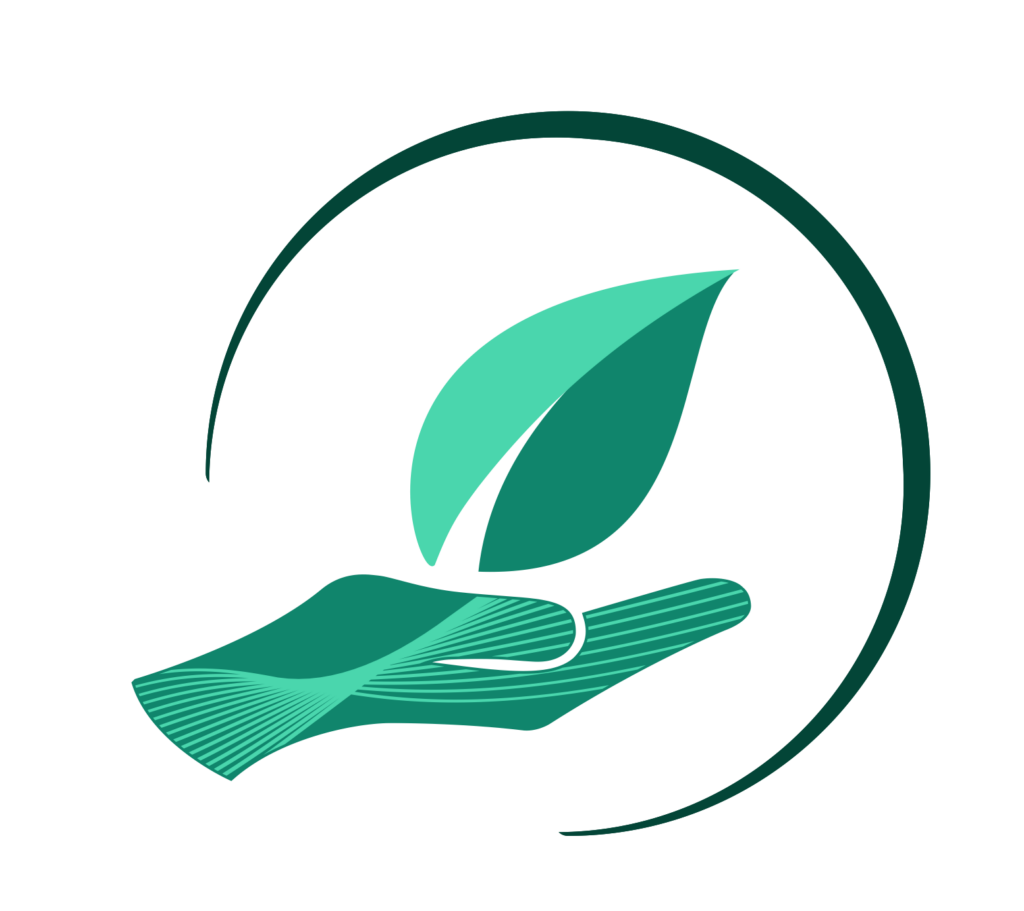
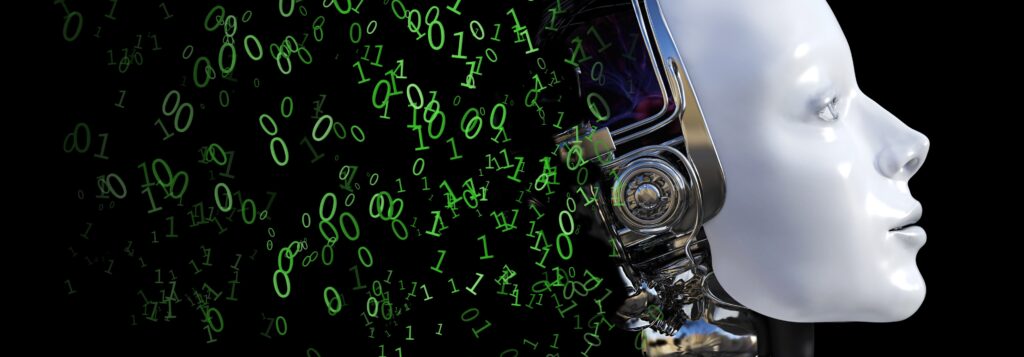
AI Ethics and Issues of Responsibility
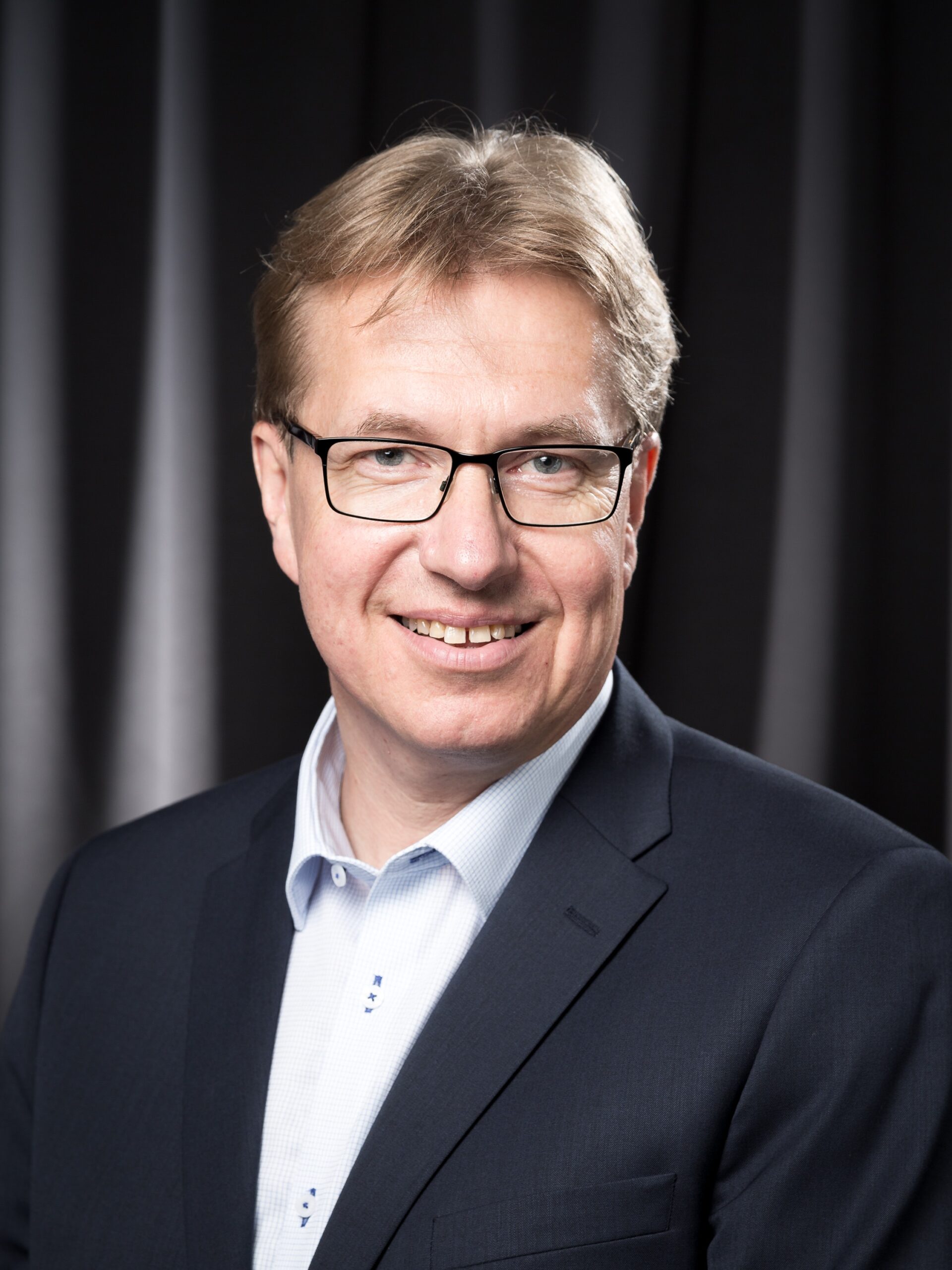
Juha Räikkä
Prof.
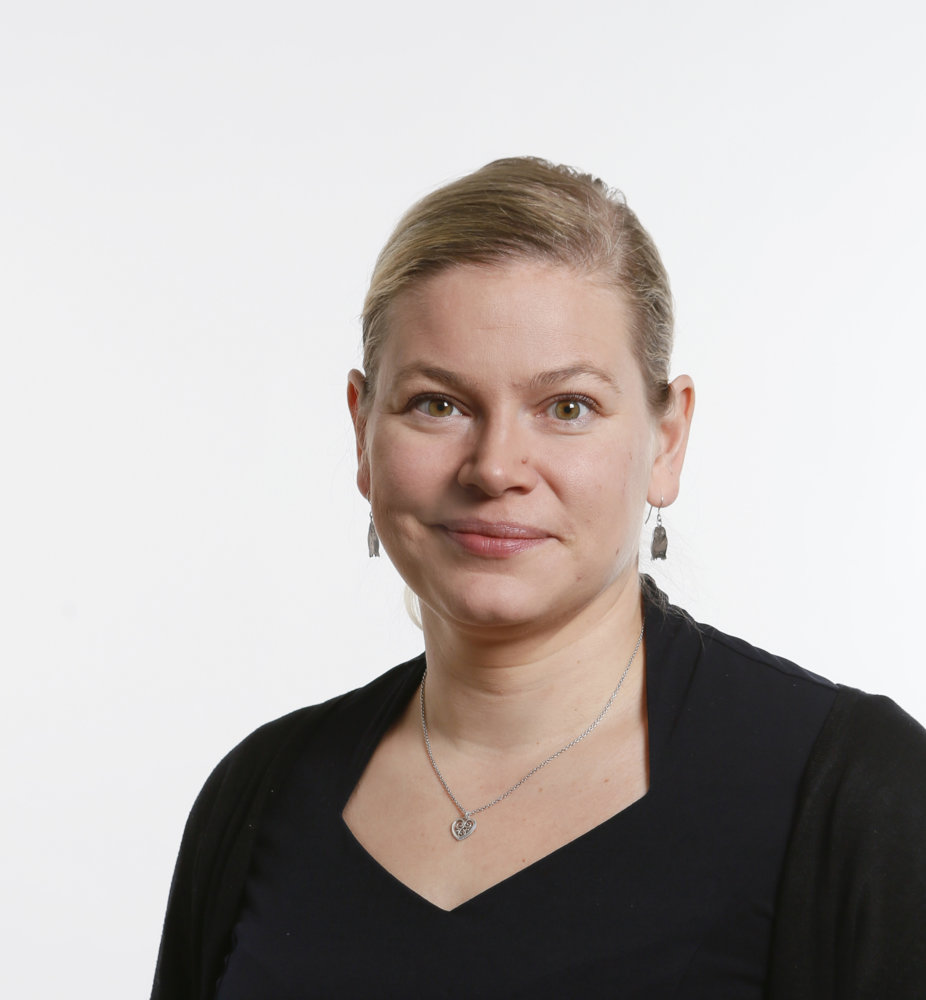
Susanne Uusitalo
Senior Researcher
Group leaders (PIs) Prof. Juha Räikkä and Senior Researcher Susanne Uusitalo
Central to the present group are questions such as whether codes of professional ethics can handle AI ethical problems exhaustively, would it be possible to include moral competency in autonomous AI agent architectures, and who is responsible and accountable of the AI-related actions and their implications. Ethical analysis is required on the role moral sentiments in responsibility attributions toward AI-agents. If we blamed them as we blame human beings, would it mean that they are responsible in the similar manner as human beings are? Or can the responsibility attributions be mistaken even if we are not mistaken on factual matters? Also, will the machine-human hybrid agents constitute an exception or exemption?
Potential dissertation topics:
Responsibility and AI
Who is responsible for AI?
Agency and AI
Does AI actually do anything?
Blameworthiness and AI
If someone refrains from using AI even when they had reason to, are they acting in way that should be blamed?
AI and democracy
AI technologies can be disruptive technology for democracy.
AI and fairness as an ethical concept
Keywords: Responsibility, agency, justice (equality and fairness), blame, democracy
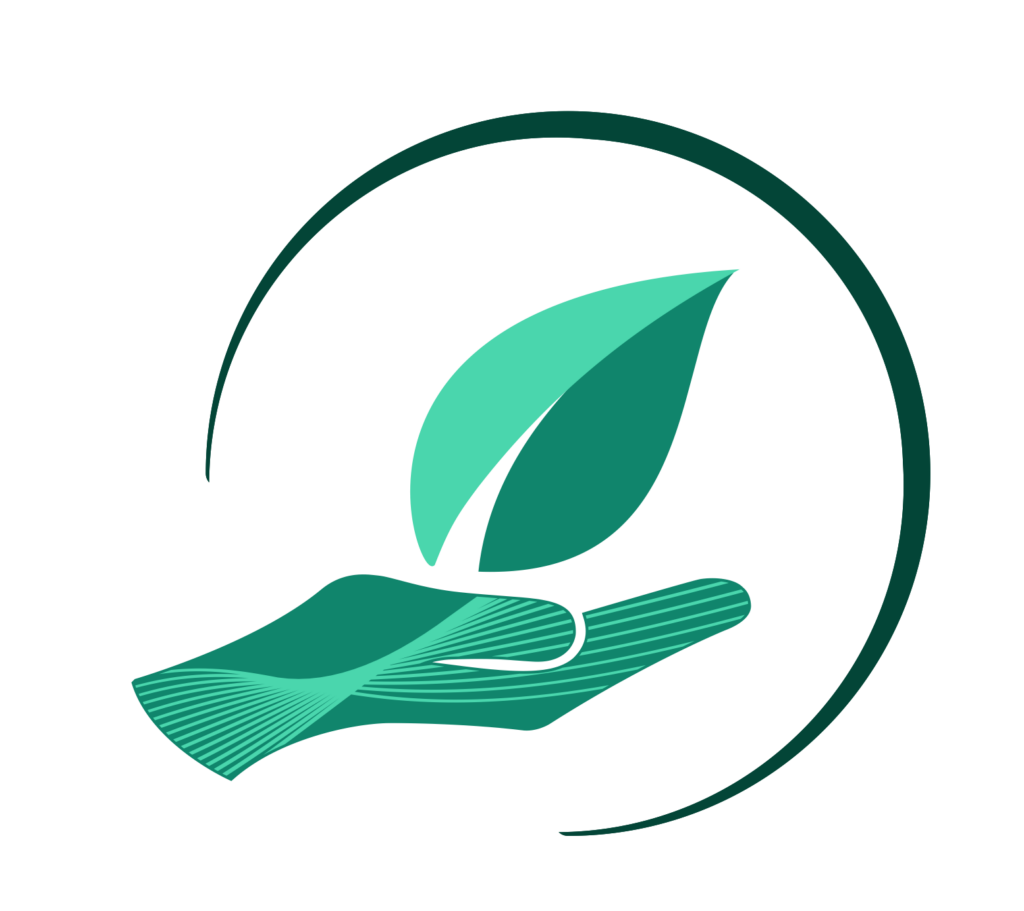
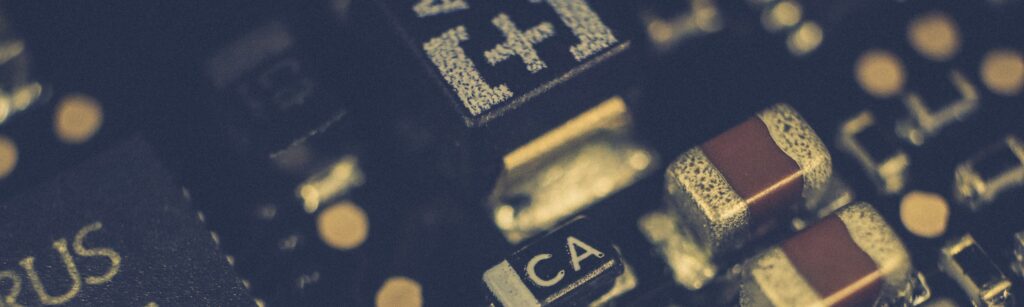
Regulating AI
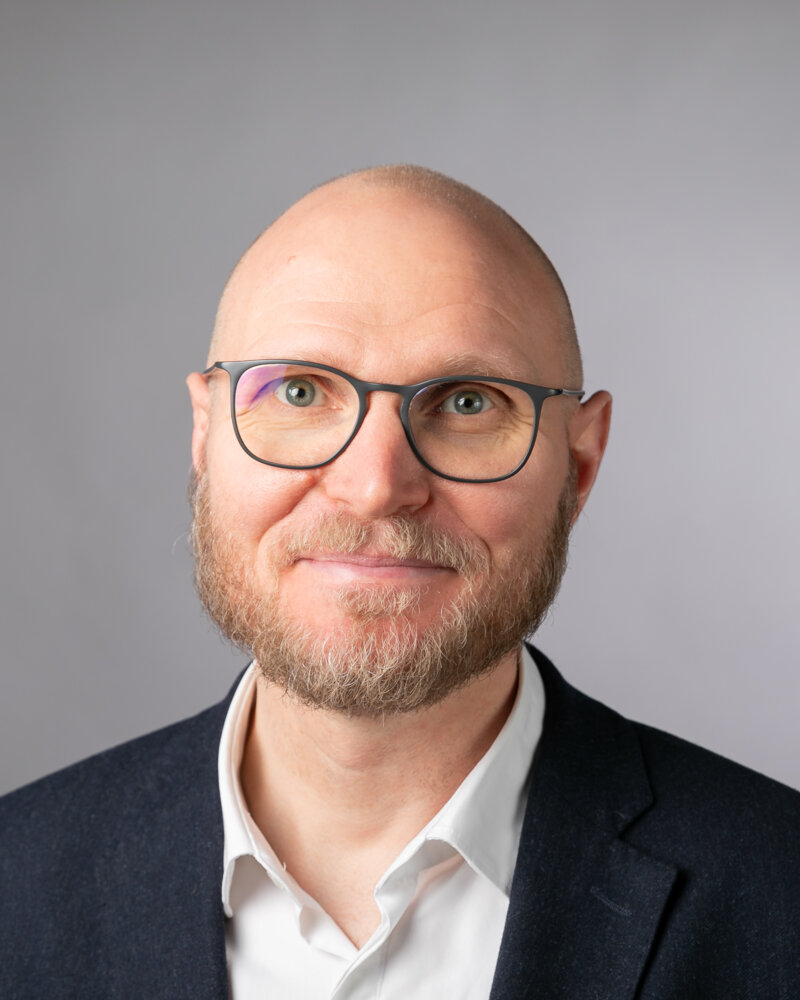
Mika Viljanen
Prof.
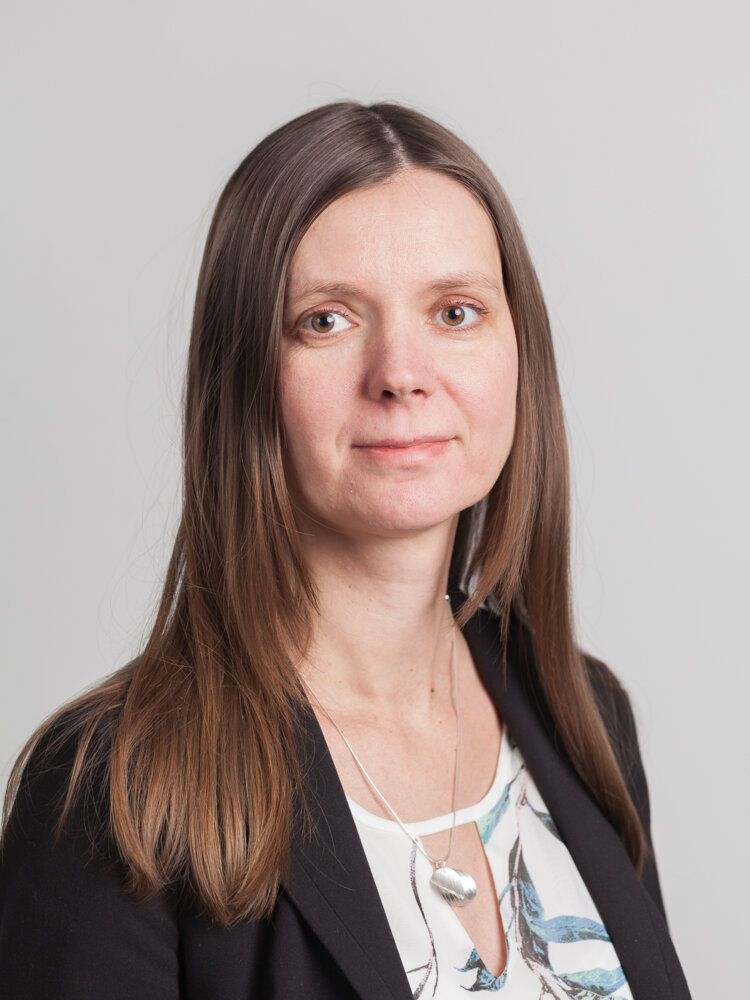
Annika Rosin
Asst. Prof.
Group leaders (PIs) Prof. Mika Viljanen and Asst. Prof. Annika Rosin
The group focuses on regulatory strategy approaches to AI regulation. The group builds its analysis on solid knowledge of existing state-of-the-art of AI and its possible future trajectories. Understanding of AI features informs the groups’ work to assess existing and proposed regulatory initiatives and developing possible regulatory approaches to harm creation. The group has focused on analysing AI-related harm generation dynamics, existing EU regulatory strategies, and robot regulation strategies. The group has also analysed the use of AI in employment context including in recruitment, direction and evaluation of work performance and termination of employment relationships.
Potential dissertation topics:
LLMs in the AI Act.
AI Act compliance for medical devices.
LLM-based decision-making, justifications and legitimacy.
Validating and testing of LLMs under the AI Act.
Automatic monitoring and decision-making in employment relationships.
Using high-risk AI systems in employment.
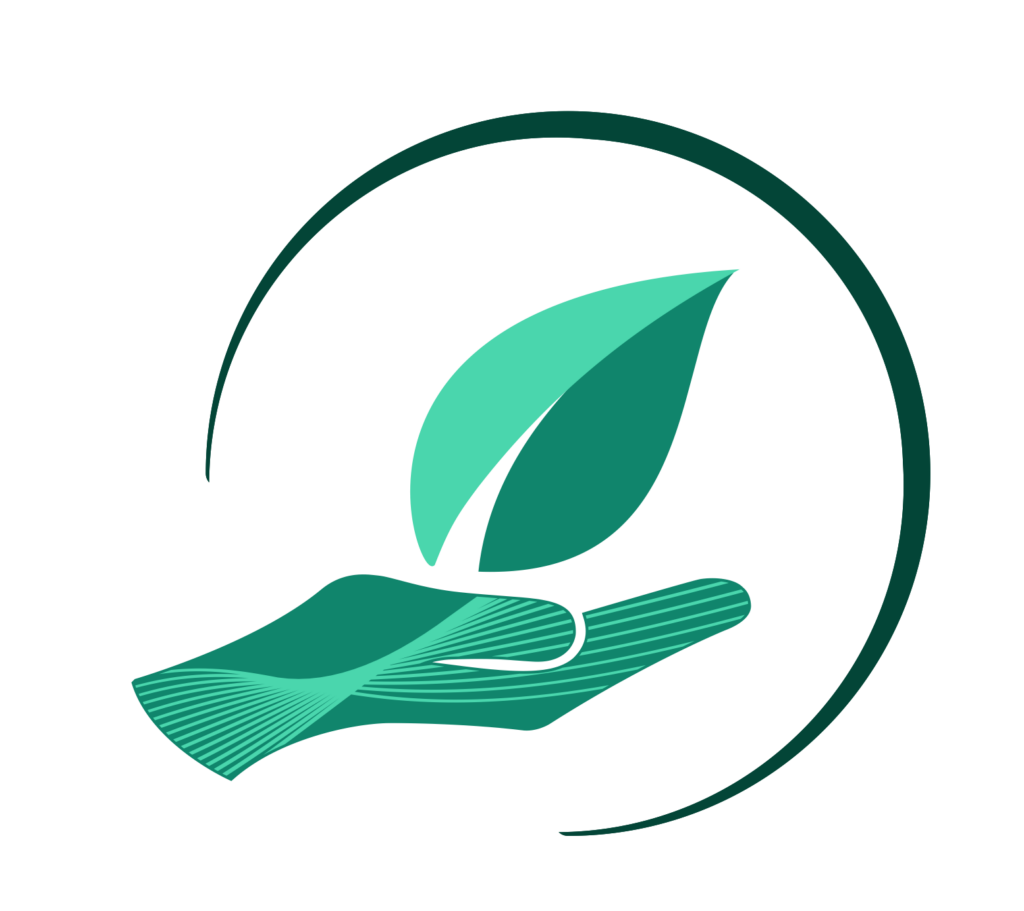
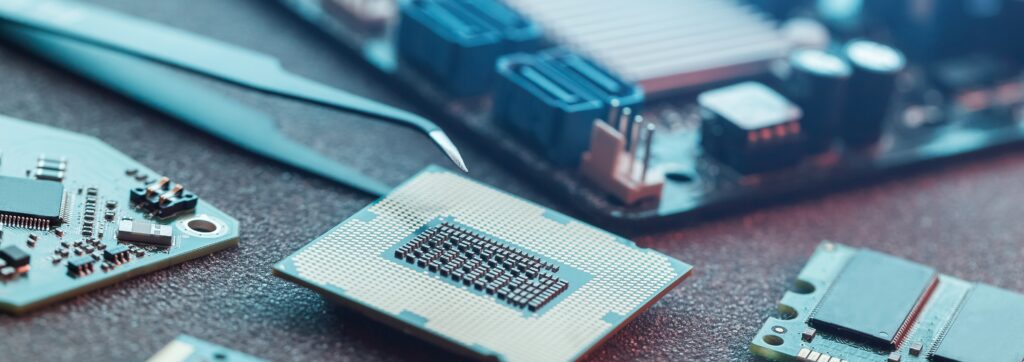
Materials Informatics Laboratory
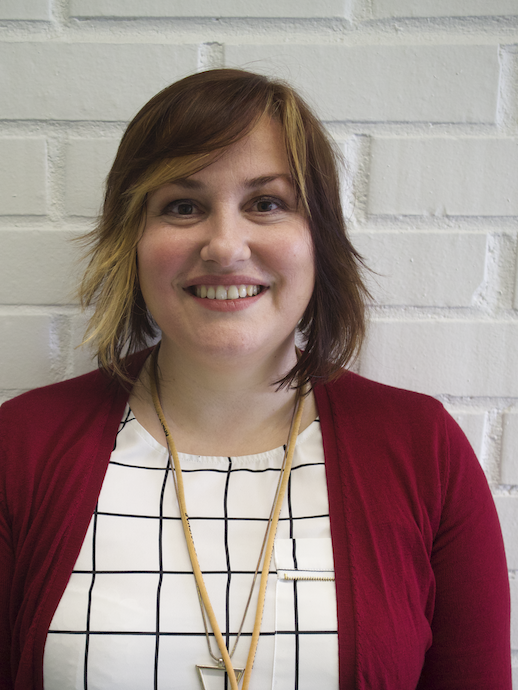
Milica Todorović
Asst. Prof
Group leader (PI) Asst. Prof. Milica Todorović
The research group combines AI and data science with materials datasets to optimise the functional properties of materials and boost their performance in technological devices. We typically deploy supervised learning to map materials processing conditions or structure to their functional properties, so that we may infer which materials design choices produce the optimal solution. We also develop probabilistic active learning methodology for materials science research and AI-guided experimentation. This allows us to integrate explainable, trustworthy, and uncertainty-aware AI into our workflows for materials discovery. Recently, our focus is increasingly shifting to multi-fidelity and multi-modal AI, and we are keen to merge information from text (NLP), images and scientific expertise (human-in-the-loop) with numerical models. We promote high quality data digitalisation and consult on AI-driven materials solutions for both academic and industrial partners.
Potential dissertation topics:
Natural language processing for information extraction in materials research.
Integrating scientific expertise into AI models for human-informed materials design.
Exploiting different sources of information in multi-fidelity AI models of materials.
Keywords: probabilistic AI, active learning, deep learning, multi-modal approaches, sustainable materials
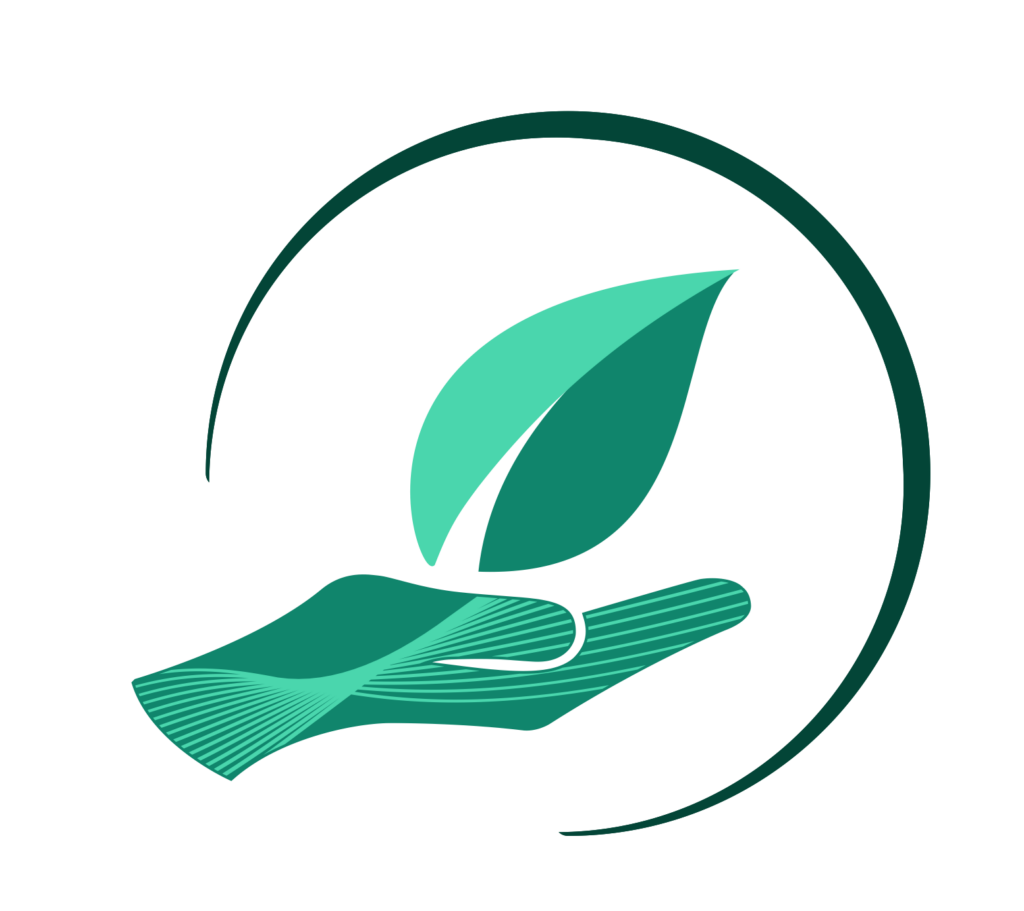
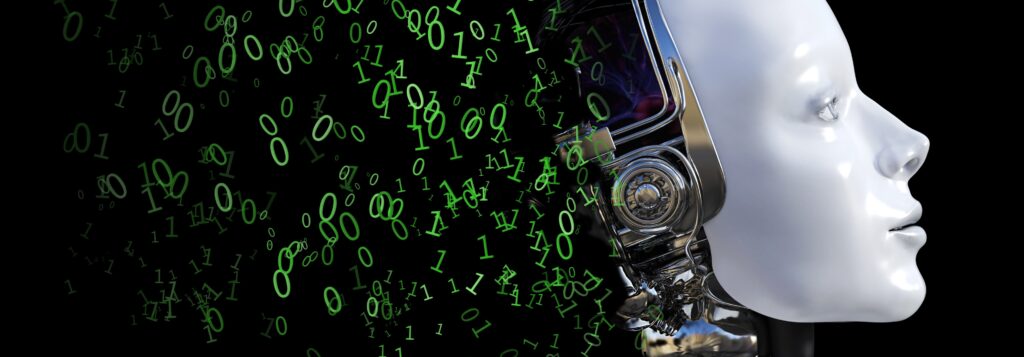
Materials in Health Technology
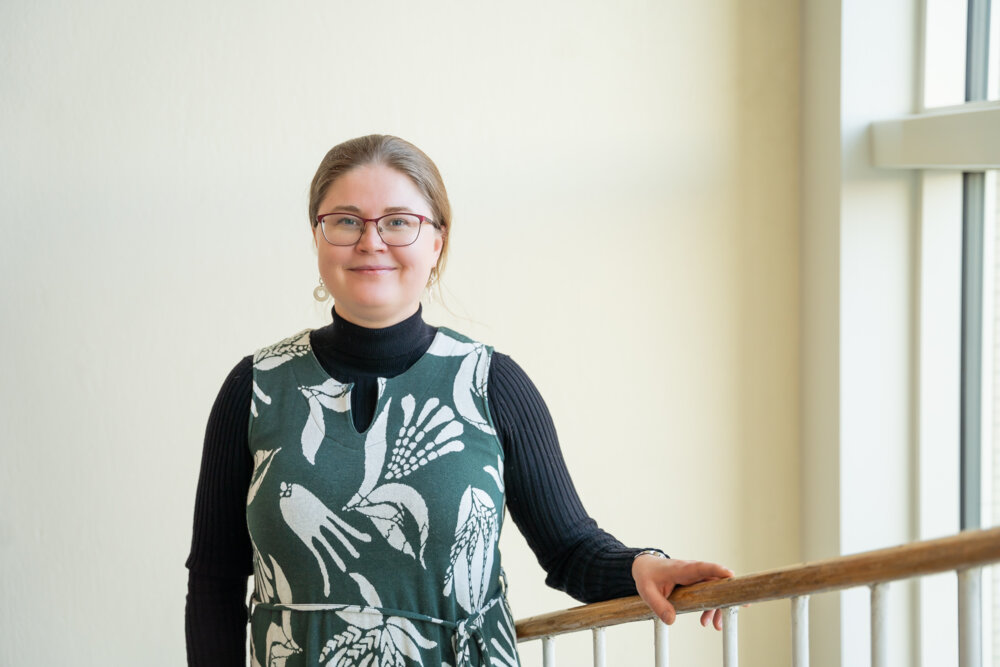
Emilia Peltola
Prof.
Group leader (PI) Assoc. Prof. Emilia Peltola
For the Materials in Health Technology Group, the research focus is in understanding the interface of materials in biomedical applications. Our goal is to enhance sensor performance in biomedical applications through a data-driven approach, strategically designing custom surfaces. We bridge the experimental-computational gap by implementing high-throughput in situ experiments and leveraging machine learning to categorize and prioritize data. This allows us to pinpoint key parameters connecting electrode surface chemistry to electrocatalytic properties and biocompatibility. Our methodology includes proposing experiments and simulations for maximum parameter information.
Potential dissertation topics:
Material Discovery: Machine learning algorithms can screen and predict environmentally friendly materials as alternatives to traditional toxic reagents in nanoparticle synthesis
Predicting synthesis outcomes: Predictive AI models can forecast the morphological and chemical characteristics of nanoparticles synthesized with various alternative chemicals, helping scientists select the safest and most efficient synthesis routes early in the research process.
AI-driven design of advanced electrodes for optimized electrocatalysis and biocompatibility.
Keywords: Sensors, experimental-computational gap, biomedical applications, nanoparticles
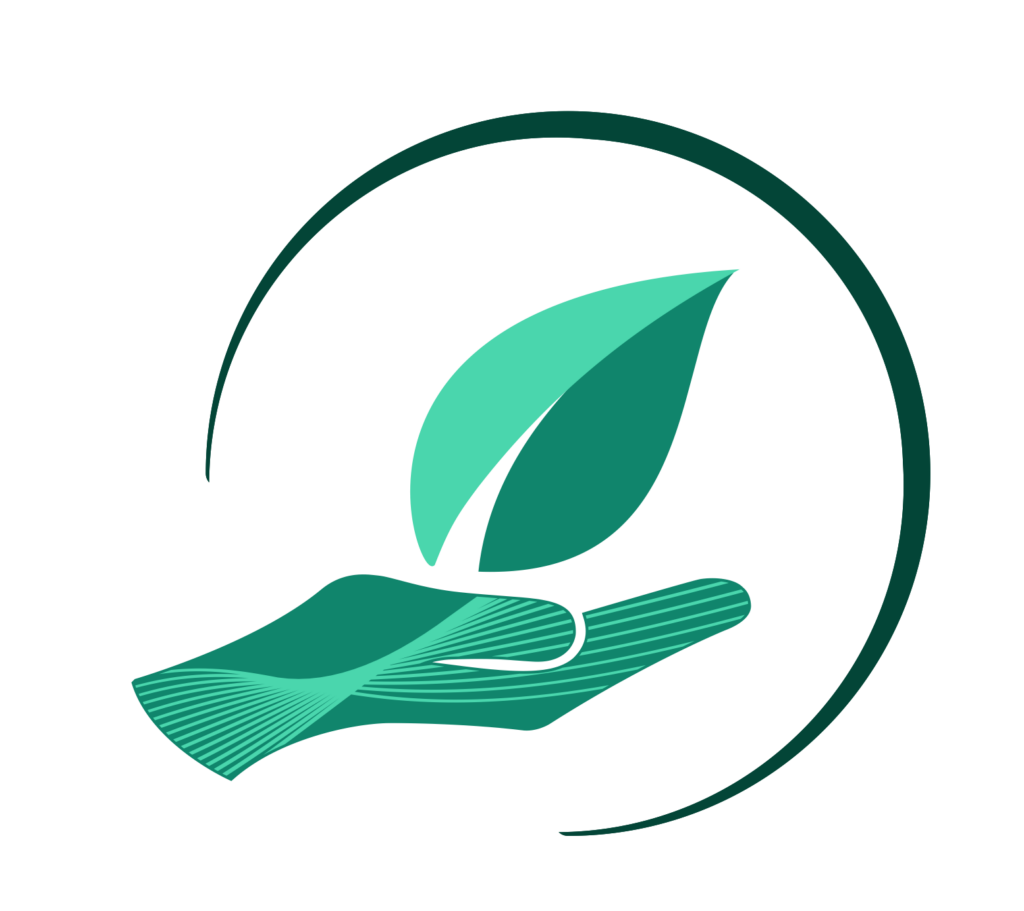
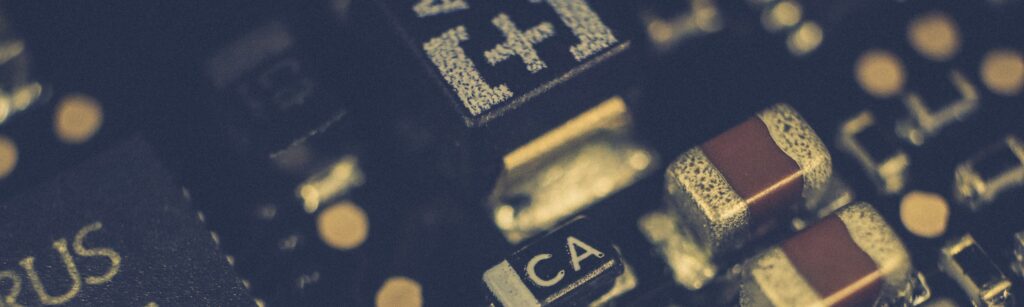
Algorithmics and Computational Intelligence (ACI) Laboratory
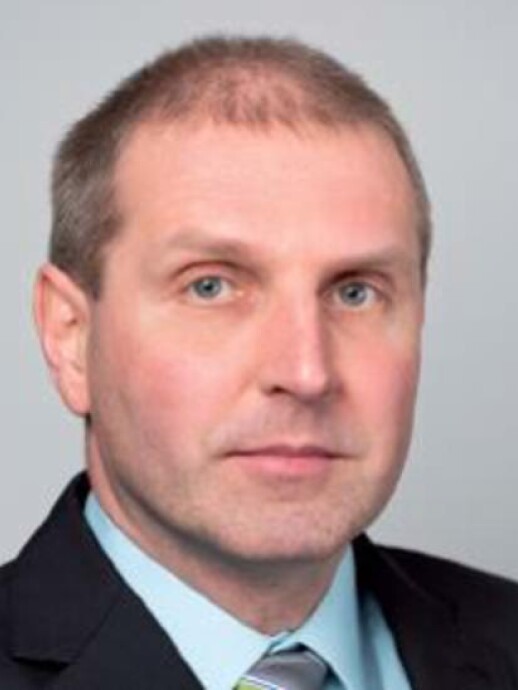
Jukka Heikkonen
Prof.
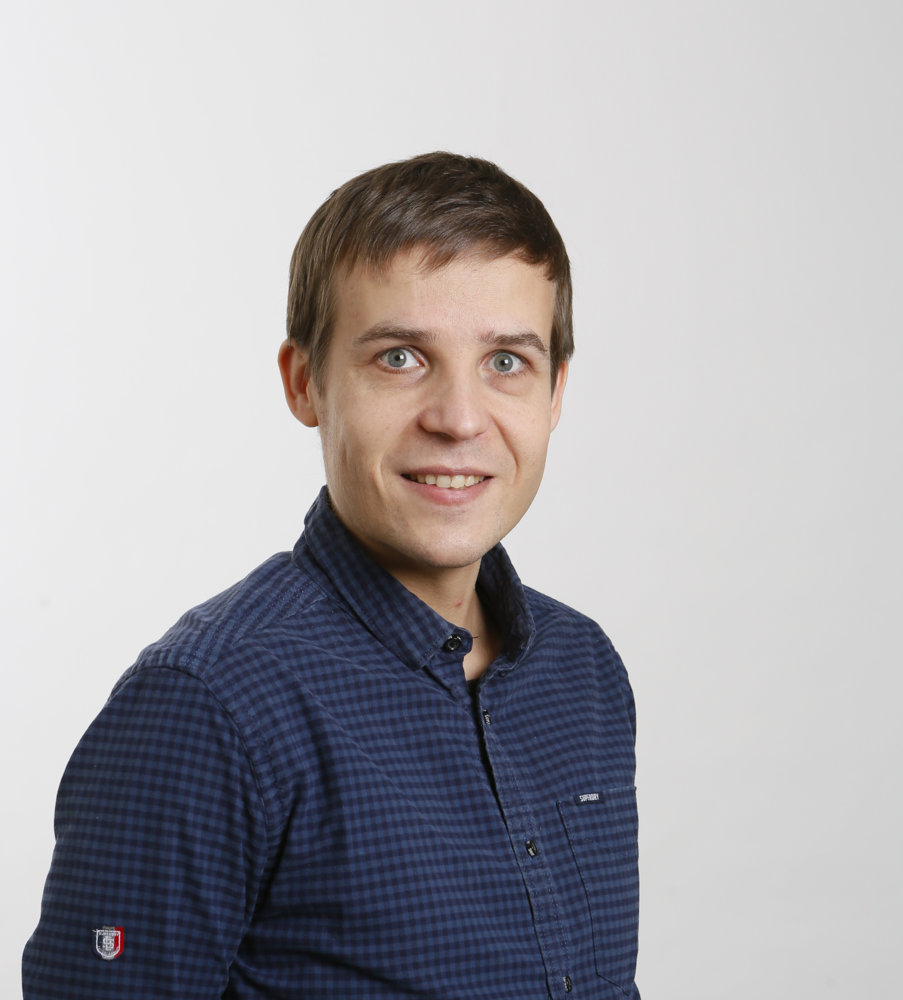
Tapio Pahikkala
Prof.
Group leaders (PIs) Profs. Jukka Heikkonen and Tapio Pahikkala
The research group is focusing on developing data analytics and machine learning techniques to address real-world challenges with sustainability and ethical considerations in mind. In the field of machine learning, our research encompasses both traditional and deep learning methodologies, placing specific emphasis on optimizing model complexity, conducting performance evaluations, and addressing issues related to robustness and scalability. Recently we have concentrated on machine learning based sensor and data fusion techniques in different domains such as autonomous systems but also when dealing with open and big data analytics especially related to various geoinformatics applications. Additionally, our research extends to algorithm design and discrete optimization, where we employ a combination of mathematical programming and heuristics to tackle complex optimization problems. We have a wide international and national collaboration network and we have actively participated in many national and international research projects, often in close partnership with industry.
Potential dissertation topics:
Trustworthy and Transparent AI in Autonomous Systems.
This research focuses on developing trustworthy and transparent AI for autonomous systems, such as self-driving vehicles, drones, or robotics. The objective is to design algorithms that intelligently integrate data from multiple heterogeneous sensors (e.g., LIDAR, radar, cameras) to improve the system’s situational awareness, supporting real-time decision-making and environmental understanding. A core aspect of the research is adopting a human-centric AI, where the algorithms are not only optimized for performance but also designed to ensure that they remain explainable, trustworthy, ethically and legally aligned. Key challenges include improving the robustness, scalability, and interpretability of the models, while ensuring transparency and accountability in autonomous decision-making processes.
Keywords: Autonomous Systems, Sensor Fusion, Situational Awareness, Interpretable AI
Smart Agriculture: AI-Driven Optimization of Feed and Nutrient Management.
This research theme focuses on applying machine learning and sensor technologies to optimize feed and nutrient management in agriculture, for instance in dairy or aquaculture industries. Automated data collection, transfer, processing, and analysis, combined with chemical quality data and AI methods, enables the modeling of various production phenomena and processes. The aim of this project is to optimize input resources to improve production outcomes in terms of quality and quantity. An example of this is the effect of feeding on the fat content and fatty acid composition of milk and fish. This research emphasizes real-world applications, where AI-driven optimization techniques not only enhance production outcomes but also minimize economic and environmental impact and resource consumption and hence increase the sustainability and profitability of food production systems.
Keywords: Precision Agriculture, AI-driven optimization, Machine Learning, Resource optimization, Sustainable food production
AI for Sustainable Energy Management in Greenhouses: Leveraging Photosynthesis Insights.
The aim is to develop AI and machine learning models that can optimize energy usage in greenhouses based on data from sensors, energy consumption, and plant growth conditions. The key challenge lies in applying knowledge of photosynthesis processes to inform energy-saving strategies while maximizing crop yield and sustainability. Research will focus on enhancing the scalability and robustness of AI models, ensuring that they are both effective in real-world applications and ethically aligned, with a focus on environmental impact and resource efficiency in sustainable greenhouse production.
Keywords: Greenhouse Optimization, Energy Efficiency, Machine Learning, Sustainability, Photosynthesis.
Personalized AI systems: Development of machine learning algorithms for training personalized AI systems.
Performance evaluation for personalized AI systems: Development of well-defined evaluation protocols and performance measures for personalized AI.
Human centric performance evaluation of AI methods: Development of well-defined evaluation protocols and performance measures for AI accounting for their fairness and algorithmic biases.
Privacy preserving data analysis: Research and development of differentially private tools and algorithms for data analytics and machine learning.
Keywords: AI Alignment, Fairness in machine learning, Performance evaluation of AI systems, Algorithmics of machine learning, Differential privacy
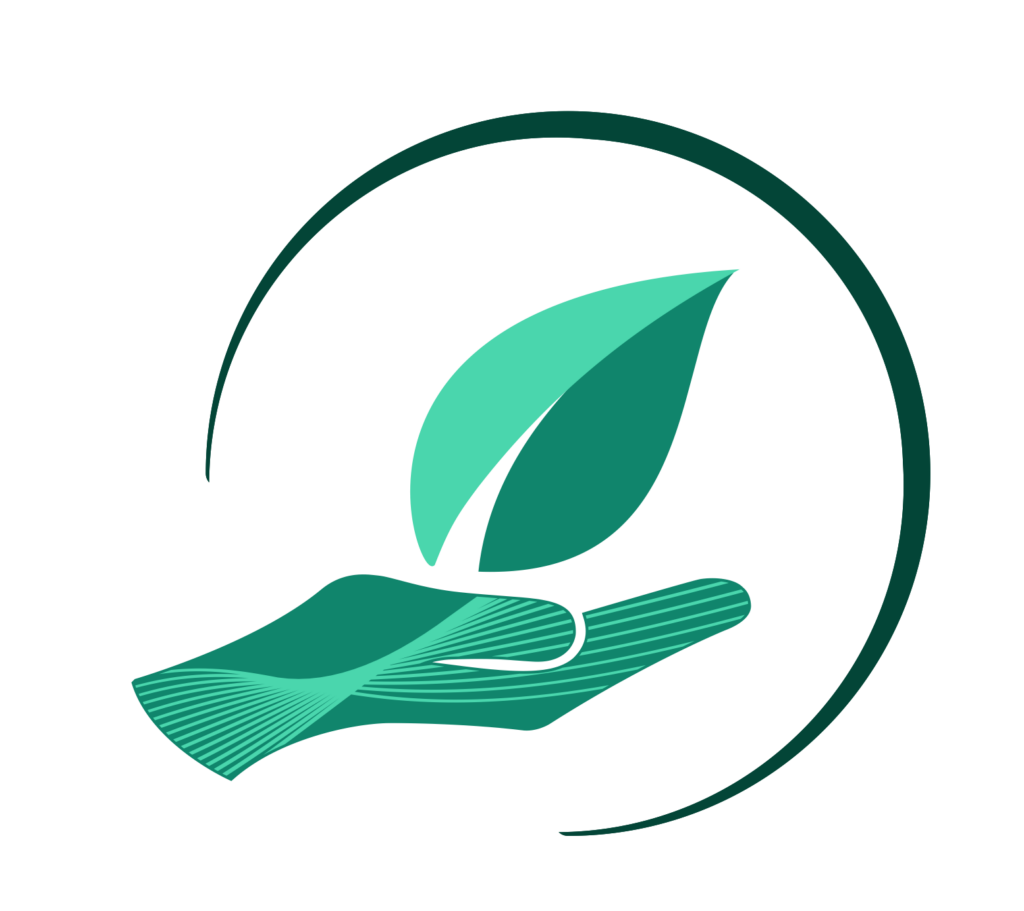
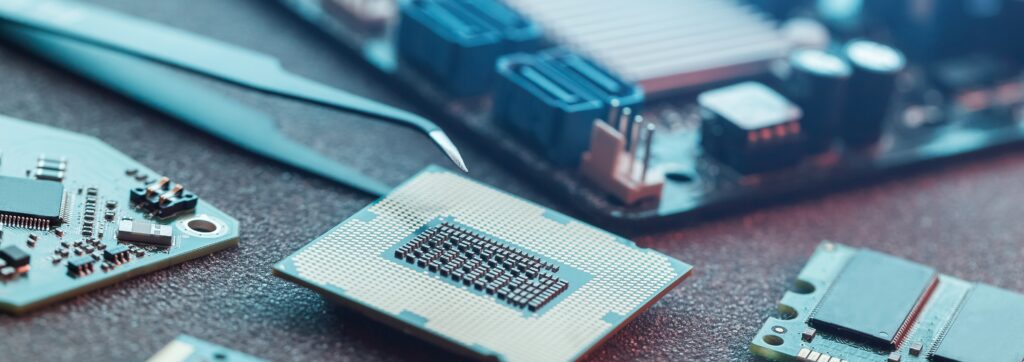
Turku Natural Language Processing Group (TurkuNLP) – Computer Science
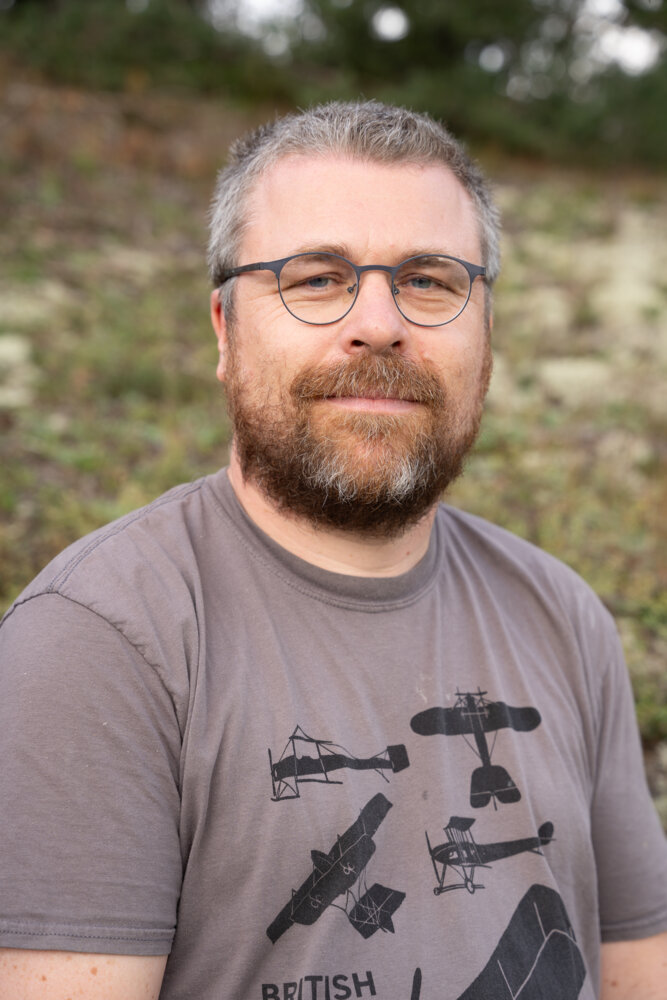
Filip Ginter
Prof.
Group leader (PI) Prof. Filip Ginter
The research group is focusing on algorithmic and computational aspects of natural language processing (NLP), one of the cornerstones of modern AI. Our research is centered around machine learning for NLP, in combination with work at a very large-scale corpora, regularly working with collections in the 100+ billion word range. Our most recent work includes training generative large language models in a high-performance computing environment, cross-lingual meaning models in historical language corpora and other noisy datasets, as well as the development of core language technology for Finnish and numerous other languages. By its nature, our research involves topics from deep neural network training to highly scalable algorithms for indexing and matching meaning across languages. We make extensive use of the national computing resources and have been selected for piloting the two most recent generations of GPU-accelerated supercomputers, including LUMI, the largest European supercomputer.
LLM-driven information extraction from web-scale corpora: Methods for efficient application of LLMs to information extraction and document categorization tasks at a very large scale. These may include research in efficient inference methodology in the technical sense, as well as applications of LLMs in specific tasks of interest.
Potential dissertation topics:
Curriculum learning in LLM training:
Methods for data-driven selection of LLM training examples so as to optimize training time and resulting model quality with especial focus on highly multilingual settings.
NLP on noisy data:
NLP methods to utilize and standardize corpora that are affected by noise, especially OCR artefacts in historical documents. These may include both LLM-driven error correction as well as embedding and retrieval methods resilient to noise.
User-guided document embeddings:
Methods for induction of flexible, user-guided document embeddings that take into account or disregard specific aspects of meaning. Large-scale applications of such methods in document retrieval and retrieval-augmented generation.
Tracing the emergence and flow of ideas in multilingual corpora:
Methods for identifying and tracing passages of related meaning in very large multilingual text collections, tracing the development and mutual interaction of ideas especially in but not limited to historical texts.
Text-based explainability:
Methods for text-based explainable NLP/AI, moving beyond feature heatmap approaches.
Keywords: NLP, large language models, large corpora, deep learning, multilingual methods
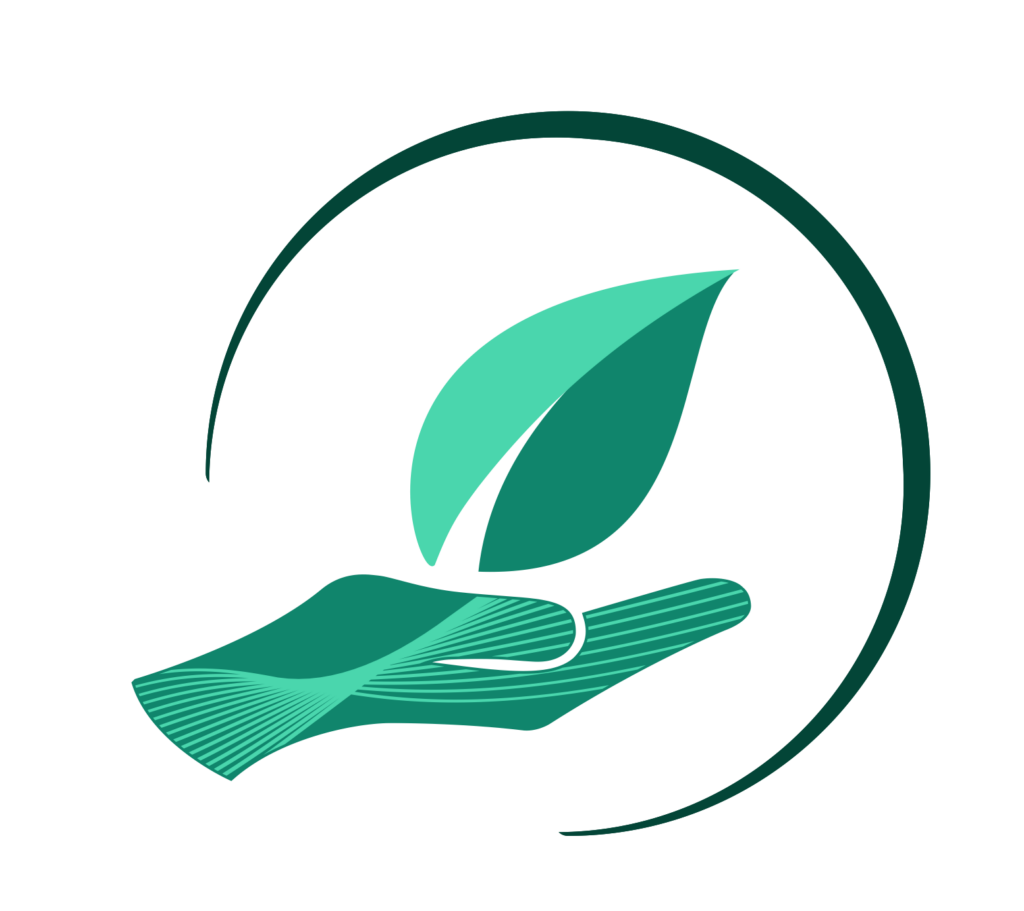
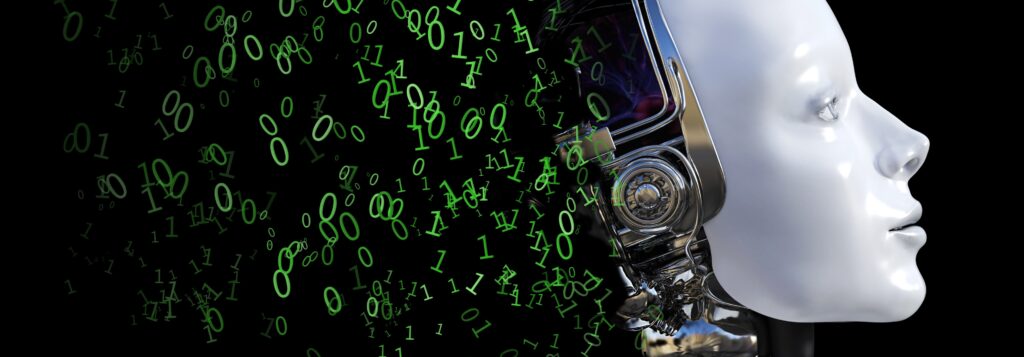
Turku Natural Language Processing Group (TurkuNLP) – Digital Linguistics
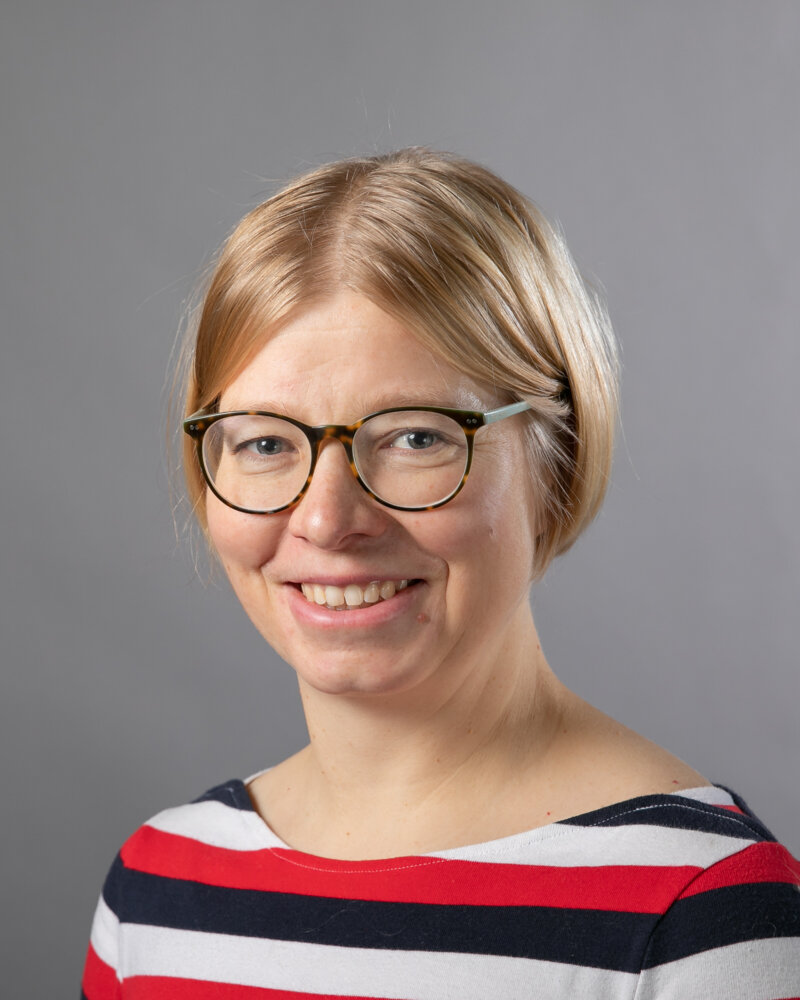
Veronika Laippala
Prof.
Group leader (PI) Prof. Veronika Laippala
The research group focuses on natural language processing (NLP) and its applications in linguistics. The core research targets the use of machine-learning based tools to examine language in large digital language datasets, typically adopting text linguistic and discourse analytic approaches and deep neural network –based methods. Additionally, a specific research focus is the development of extremely large web-based language datasets, in a tight collaboration with TurkuNLP / Computer science. Similarly, we develop together novel language technology methods for Finnish and other languages. The recent projects center around 1) text registers (genres) in massively multilingual web-scale corpora and in historical collections, 2) language use in specific settings, such as social media and crisis communication, 3) language and society, such as characteristics of parliamentary speeches and language and climate change. In all these, the to pics are approached in large born digital or digitized data and using machine learning.
Potential dissertation topics:
Tracing cross-lingual and language-specific tendencies in language use with LLMs and web-scale data.
LLMs give novel possibilities to analyze multilingual data. While typical cross-lingual comparisons in linguistics require heavy manual work, LLMs allow presenting texts in one multilingual space and computationally comparing them across languages. How do texts such as social media or news articles vary across languages? What similarities and differences can we find? How well do the models perform in this task? What limitations can we find? The project can either focus on a small group of texts and languages or cover a large set of them.
From discrete to continuous – modeling web registers in a continuous space in large-scale multilingual web data.
Traditionally, register categories like news articles, how-to pages or opinion pieces have been considered as discrete classes. However, recent studies on small-scale datasets in English have shown that registers could be better modeled as continuous, with documents mixing features from several classes. This applies in particular to large-scale web data with extreme variation. How can AI be used model these continuous spaces? What axes of variation do we find, and how do they vary across languages?
Minority languages on the web.
Massive web-crawls and AI methods bring new possibilities to the study of minority languages. Relying on AI-based methods and focusing on one or several minority languages, this project would analyze minority language use in web-scale data. What kinds of differences do these bear when compared to majority languages or dominant varieties of the studied languages?
Comparing LLMs and statistical methods in corpus linguistics.
Corpus linguistics relies heavily on statistical methods and very standardized analytical approaches. Although the potential of LLMs is widely known, the field would benefit from systematic comparisons between the approaches and a better understanding of the possibilities and limits of LLMs for linguistic analysis. This project would target this gap.
Keywords: natural language processing, digital linguistics, web data, crosslingual learning, web registers
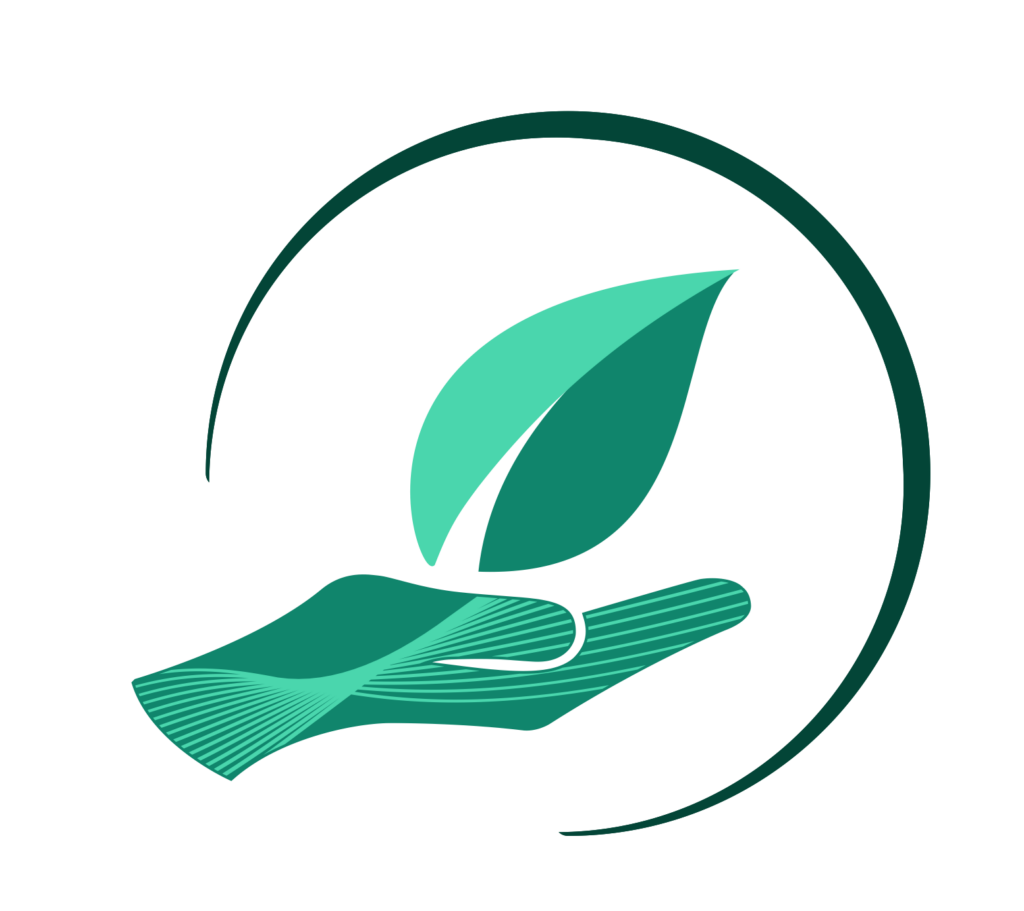
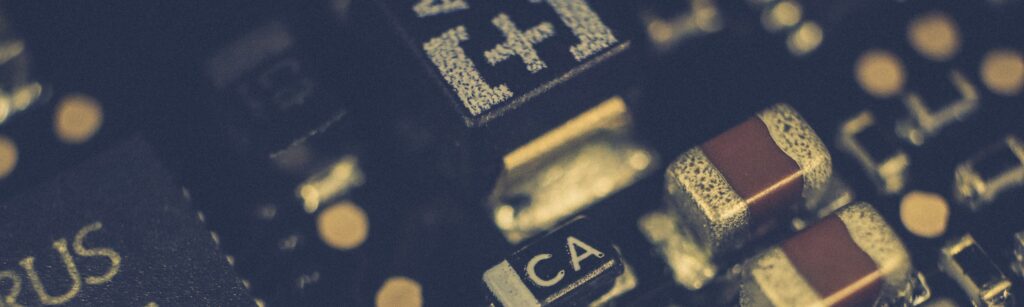
Cyber Security Engineering
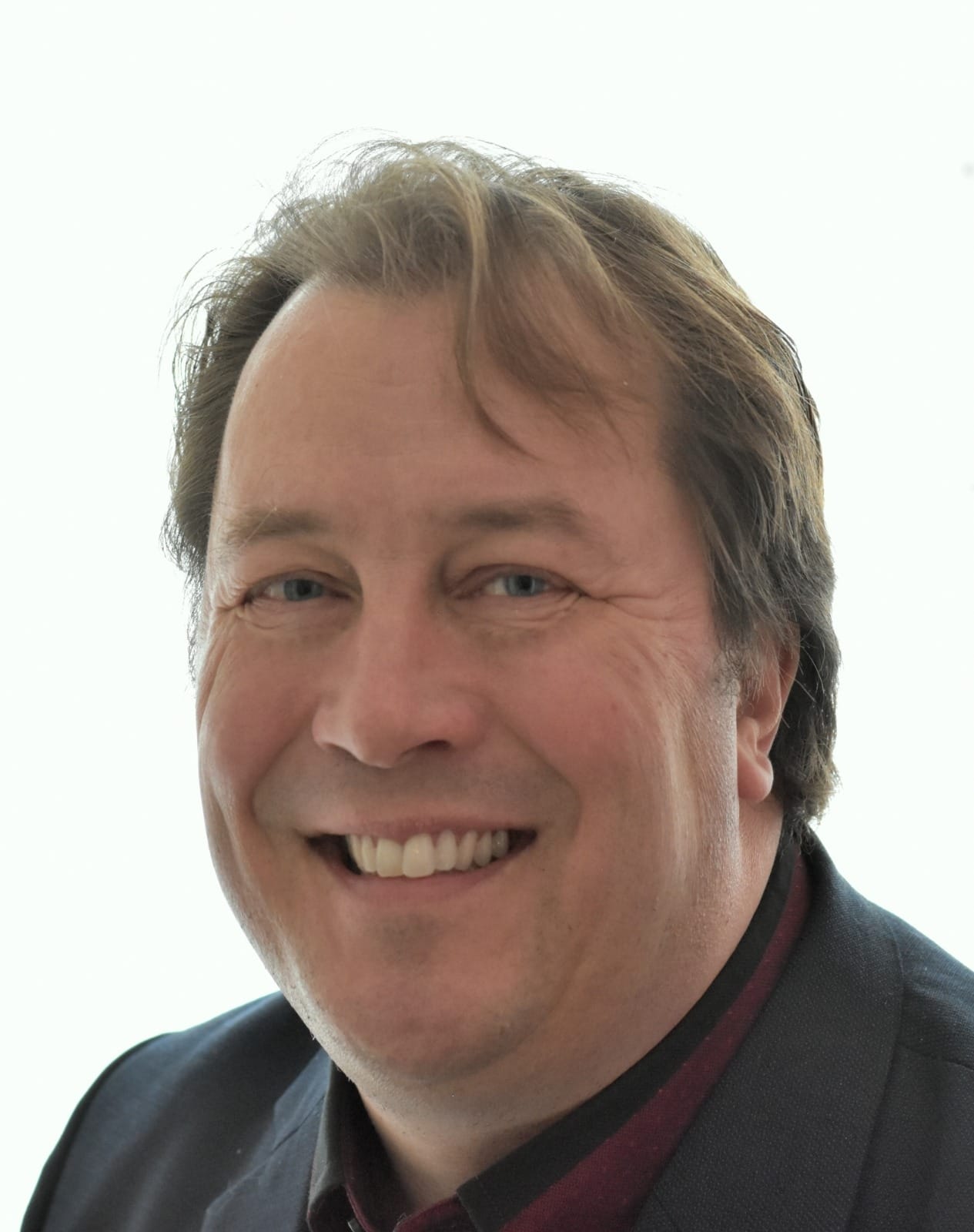
Seppo Virtanen
Prof.
Group leader (PI) Prof. Seppo Virtanen
The research group conducts research and develops technologies at all abstraction levels and key application domains of networked systems, targeting future technologies needed in building a secure digital society. The group’s research focuses on three main areas: 1) application-domain specific cyber security: ensuring the security, privacy and safety of networks, communication and data from short range IoT to global internetworking, in key digital application domains such as e-Health and well-being, autonomous systems and AI, and smart cities and environments; 2) cyber security technology and assessment: cyber security technologies and assessment methods for future networked systems; new detection technologies for network security solutions; the application of AI and LLMs to cyber security; methods for assessing and predicting the security levels of deployed networks; systems with security, privacy and safety considered as elements to be built in; and 3) cyber security for the society and the individual: addressing the human factor in cyber security to prevent the potential massive personal and societal damage arising from the threats and risks in the wide-spread adoption of new technologies (smart environments, e-Health, e-voting) by individuals and organizations; securing the human side of digital transformation.
Potential dissertation topics:
Human-Centric AI for Cybersecurity Incident Response and Threat Intelligence: This research will focus on developing real-time threat detection models to assist the security analyst in making informed decisions and speeding up threat detection and mitigation. The intersection of advanced AI capabilities and human expertise holds the potential to improve response effectiveness, reduce incident resolution times, and ultimately bolster organizational cybersecurity resilience.
Keywords: Cyber Security, Threat Intelligence, Large Language Models, Deep Neural Network, Human in Loop, Automated threat detection.
AI-Driven Threat Detection with Human-in-the-Loop to Enhance Transparency and Interpretability in Cyber Defense Systems: This research will focus on reliability and accuracy within AI systems, which are critical for fostering trust in the output generated by AI-enabled systems, particularly in the context of security incidents. Emphasizing interpretability is important, as it enables the security analysts to understand the reasoning behind the recommendations, thereby fostering trust and facilitating more informed decision-making during critical incidents.
Keywords: Cyber Security, Threat Intelligence, Large Language Models, Deep Neural Network, Human in Loop, Automated threat detection.
Generative AI powered self-securing system for critical infrastructure: The research aims to develop an autonomous, adaptive cybersecurity system capable of proactive threat detection, rapid response, and continuous learning while maintaining transparency and user trust. This will enhance the protection of critical infrastructure against evolving cyber threats while minimizing human made configuration error.
Keywords: Cyber Security, Threat Intelligence, Large Language Models, Deep Neural Network, Human in Loop, Automated threat detection.
Assurance of user privacy and security in the age of AI proliferation: This research will focus on developing technologies that protect user privacy and security while maintaining usability. Furthermore, the research will also explore how users develop trust in AI-enabled digital systems and identifying the transparency mechanisms that enhance or diminish this trust. This facilitates developing methodology for managing, understanding and mitigating forced trust issues with AI enhanced digital infrastructure.
Keywords: Trust, Security and Privacy, Forced trust, Usability, Digital Infrastructure, Artificial Intelligence.
Inclusive explainable security: This research focuses on developing frameworks, models and tools for building personalized AI security consultants that would be capable to help people coming from varying backgrounds with cyber security issues. Misaligned mental models can lead to risky behavior; thus, this research area focuses on bridging the gap between user understanding and technical realities. Vulnerable demographics such as children and the elderly face increasing challenges with rapidly developing cyber security requirements in modern society. Similarly, neurodivergent people may need tailored guidelines and support tools to navigate the cyber security maze. This may lead to inclusive designs that accommodate diverse cognitive processing styles hence improving the overall security landscape.
Keywords: Cybersecurity, inclusivity, neurodivergence, security and privacy, demographics, AI-assistant.
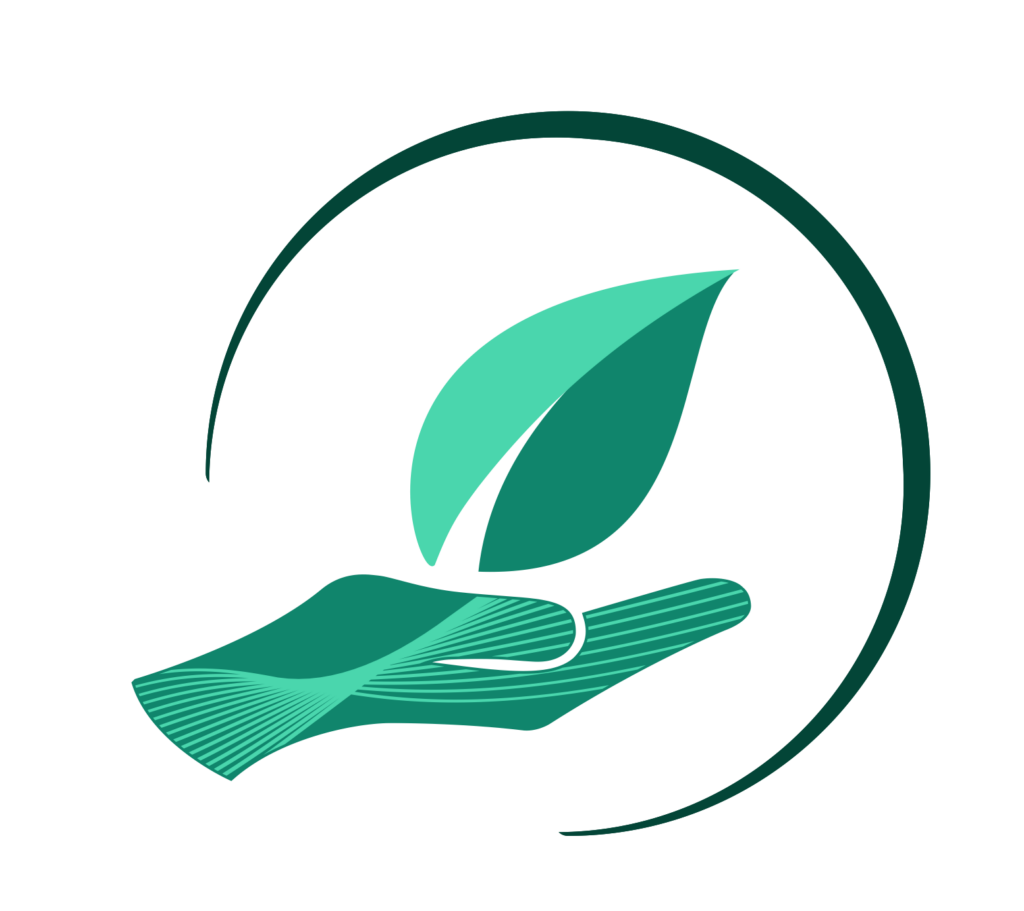
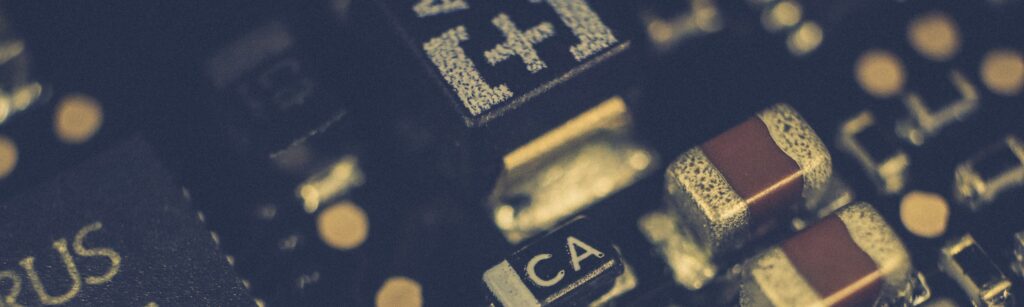
Intelligent health
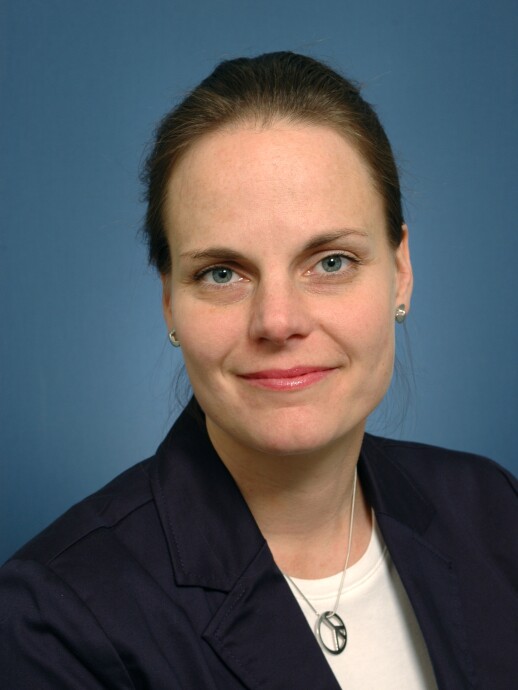
Anna Axelin
Prof.
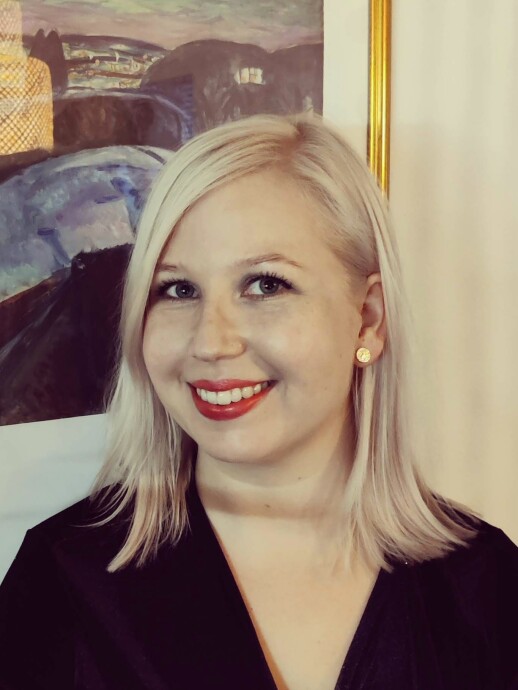
Tella Lantta
Docent
Group leaders (PIs) Prof. Anna Axelin and Docent Tella Lantta
The research group focuses on advancing the use of digital and social innovations to create value for health. We are dedicated to advancing mental health promotion and care through the development of highly personalized digital tools for early detection, prevention and care across a range of symptoms, problems and disorders. We have a strong stakeholder approach and conduct research in partnership with patients, families, professionals, healthcare organizations and companies.
Potential dissertation topics:
A Conversational Health Agent for Mental Health Promotion:
Conversational Health Agents (CHAs) have the potential to deliver personalized and effective mental health promotion, but currently lack comprehensive personalization, access to the latest health data and evidence, analytical and predictive tools, and multi-step problem solving capabilities. In this project, we will develop a personalized CHA based on self-determination theory and Large Language Models (LLM). In the next phase, it is pivotal to test the validity and feasibility of the CHA framework in real life context before its clinical evaluation as it may contain technical problems, language issues, biases, and stereotypes that can cause further problems.
Keywords: Mental health, Health promotion, Personalized care, Conversational Health Agents, Large language models